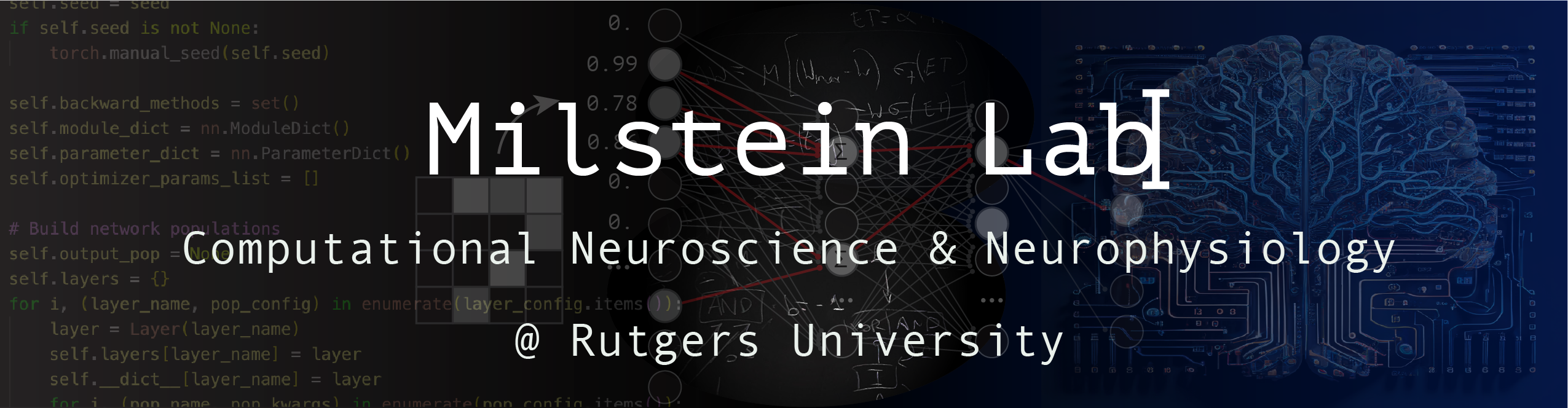
Read more about our research (including interviews with Aaron Milstein) on the Rutgers CABM lab website
Neurophysiology
Behavioral timescale synaptic plasticity (BTSP)
The recent discovery of behavioral timescale synaptic plasticity driven by dendritic calcium spikes and/or burst spiking in both hippocampal and cortical pyramidal neurons has changed the way we think about learning in the brain and inspired a new approach to understanding circuit function.
Our experimental work using in-vitro patch clamp electrophysiology aims to uncover how dendritic spikes shape BTSP-like learning in different cell types throughout the brain, and how this is disrupted in mouse models of learning disabilities.
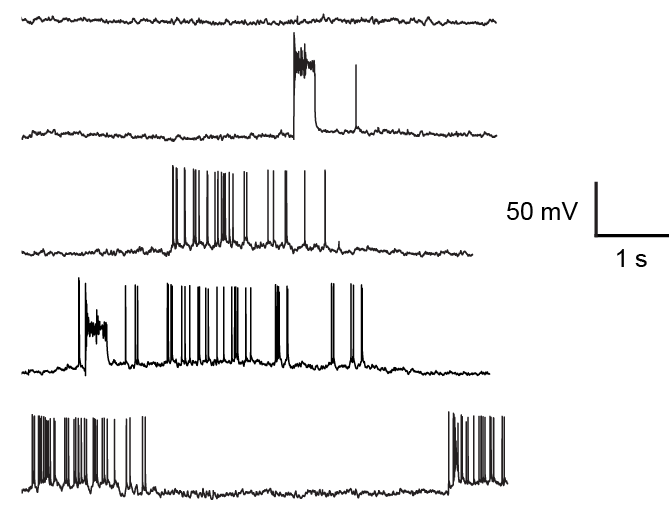
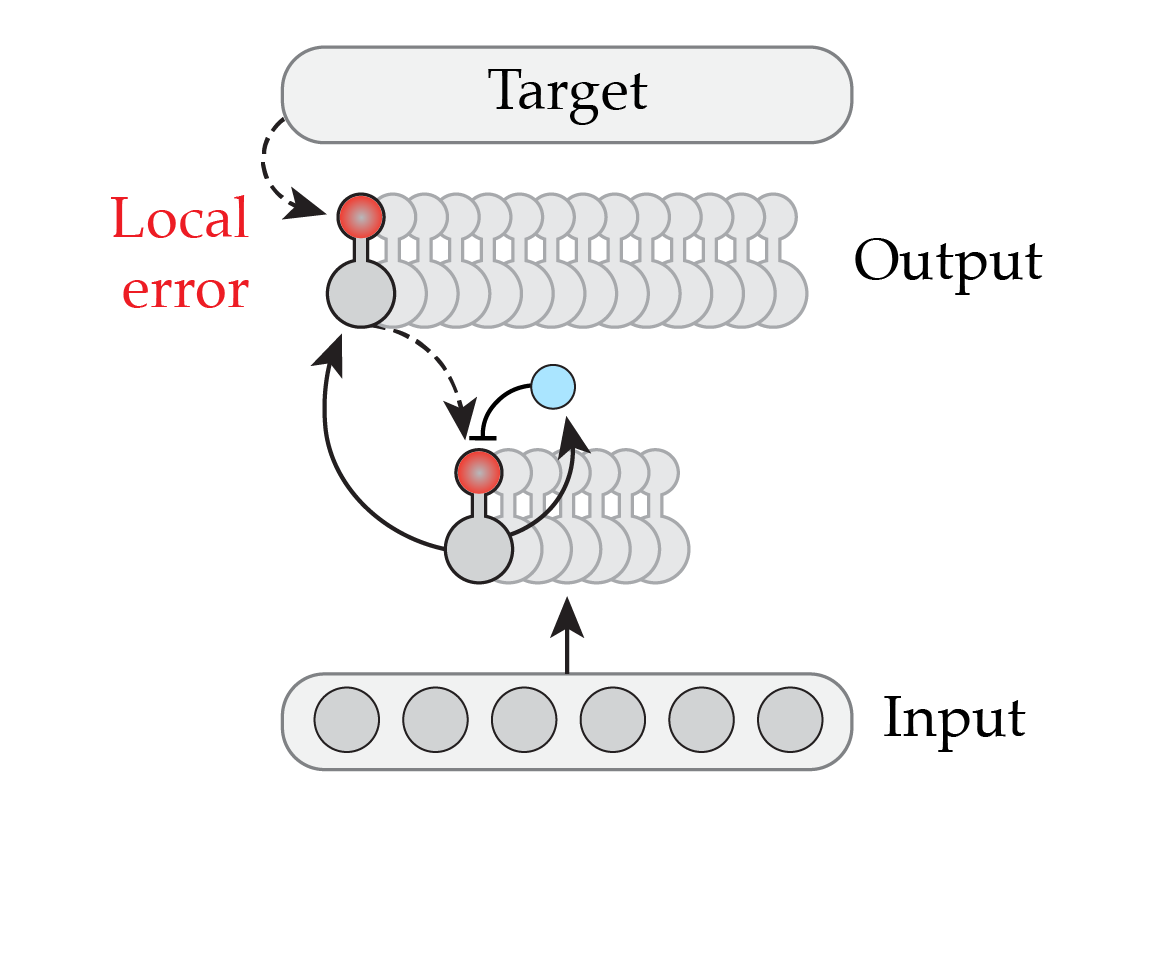
Computational Neuroscience
Biologically plausible credit assignment
We believe one of the most promising ways of understanding how the brain works is to understand how it is wired through synaptic plasticity. How do connections form and change as we learn, in a way that makes us consistently improve over time? In the field of Deep Learning this is known as the “credit assignment problem”: each synapse is updated in proportion to its contribution to a correct or incorrect decision. Effective solutions to this problem (e.g. with the backpropagation-of-error algorithm, a.k.a. backprop) have led to huge breakthroughs in AI.
While the brain is confronted with similar challenges, it’s solution(s) to credit assignment are unknown. Drawing directly from experimentally observed synaptic plasticity mechanisms (such as BTSP), we build biologically constrained neural networks with separate excitatory and inhibitory populations and realistic learning rules to explore the space of possible solutions the brain might be using.
Neuromorphic computing
Deep Learning has revolutionized AI and will have substantial implications for society, but these advancements come at great cost: the immense power consumption of GPUs running neural networks is both expensive and has serious environmental impact.
Neuromorphic computers, with biologically inspired neurons and local learning rules, stand to greatly alleviate these problems. The huge spiking neural networks in our biological brains run on less power than a lightbulb, and brain-inspired neuromorphic hardware has already shown energy efficiency orders of magnitude higher than conventional processors. However, finding effective algorithms to train neural networks running on these devices has proven a significant challenge. Part of our work is aimed at translating insights about learning mechanisms in the brain to algorithms that can operate on various types of neuromorphic hardware.
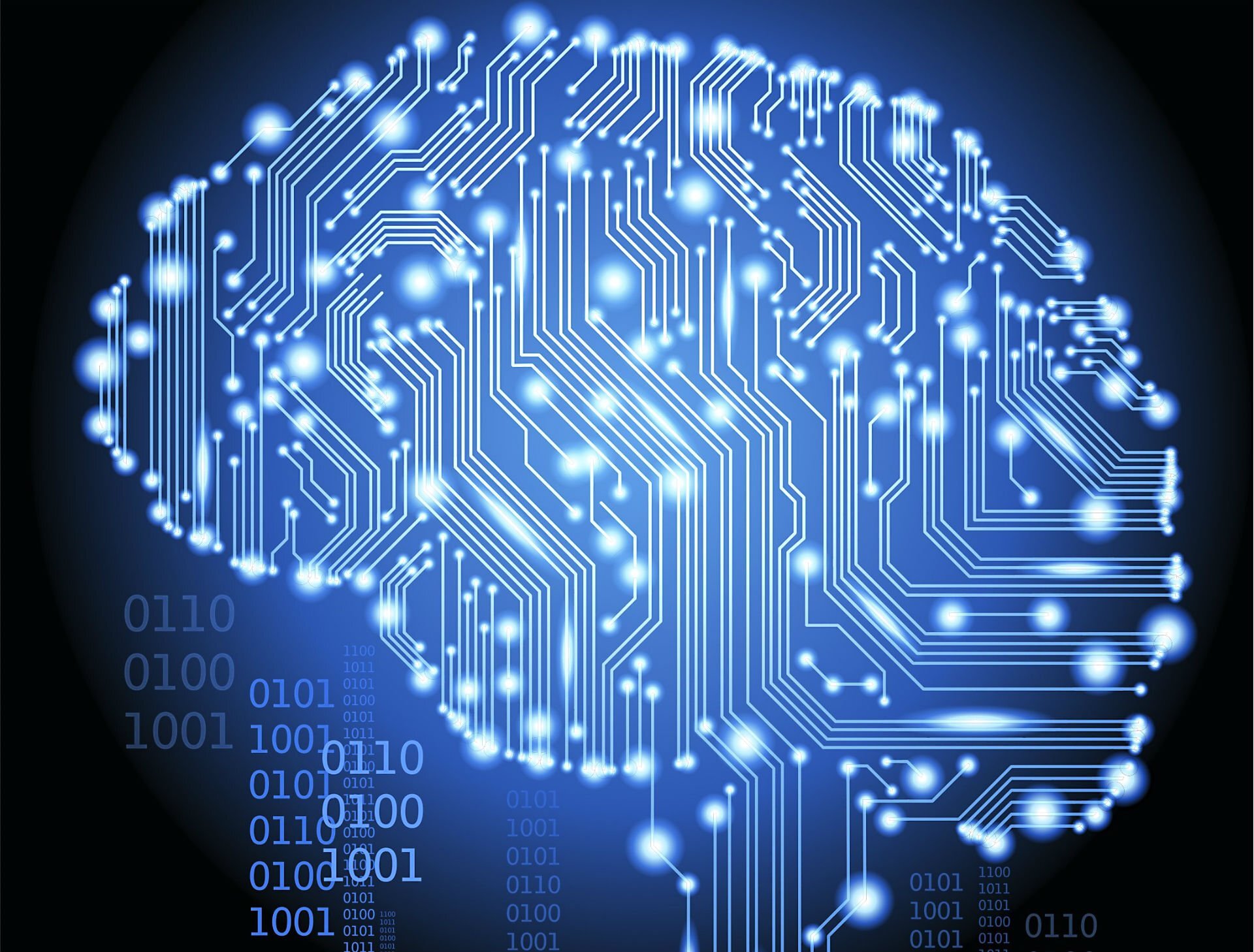
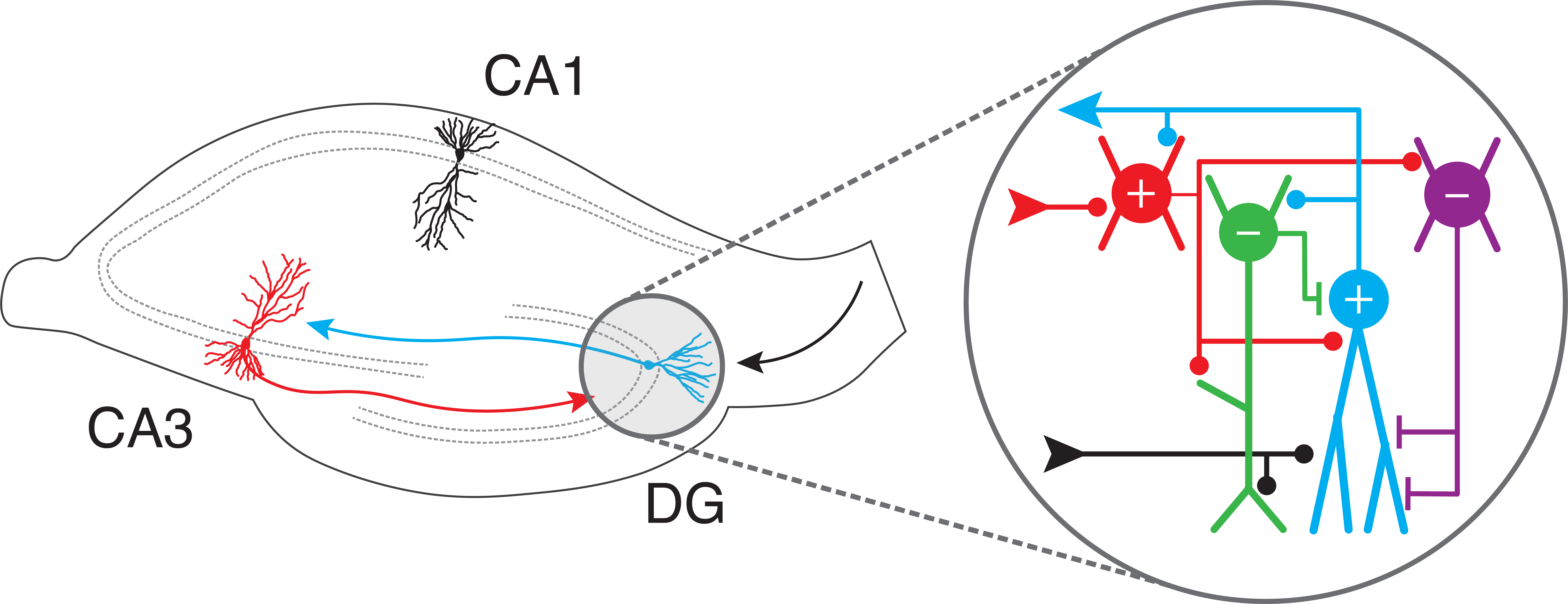
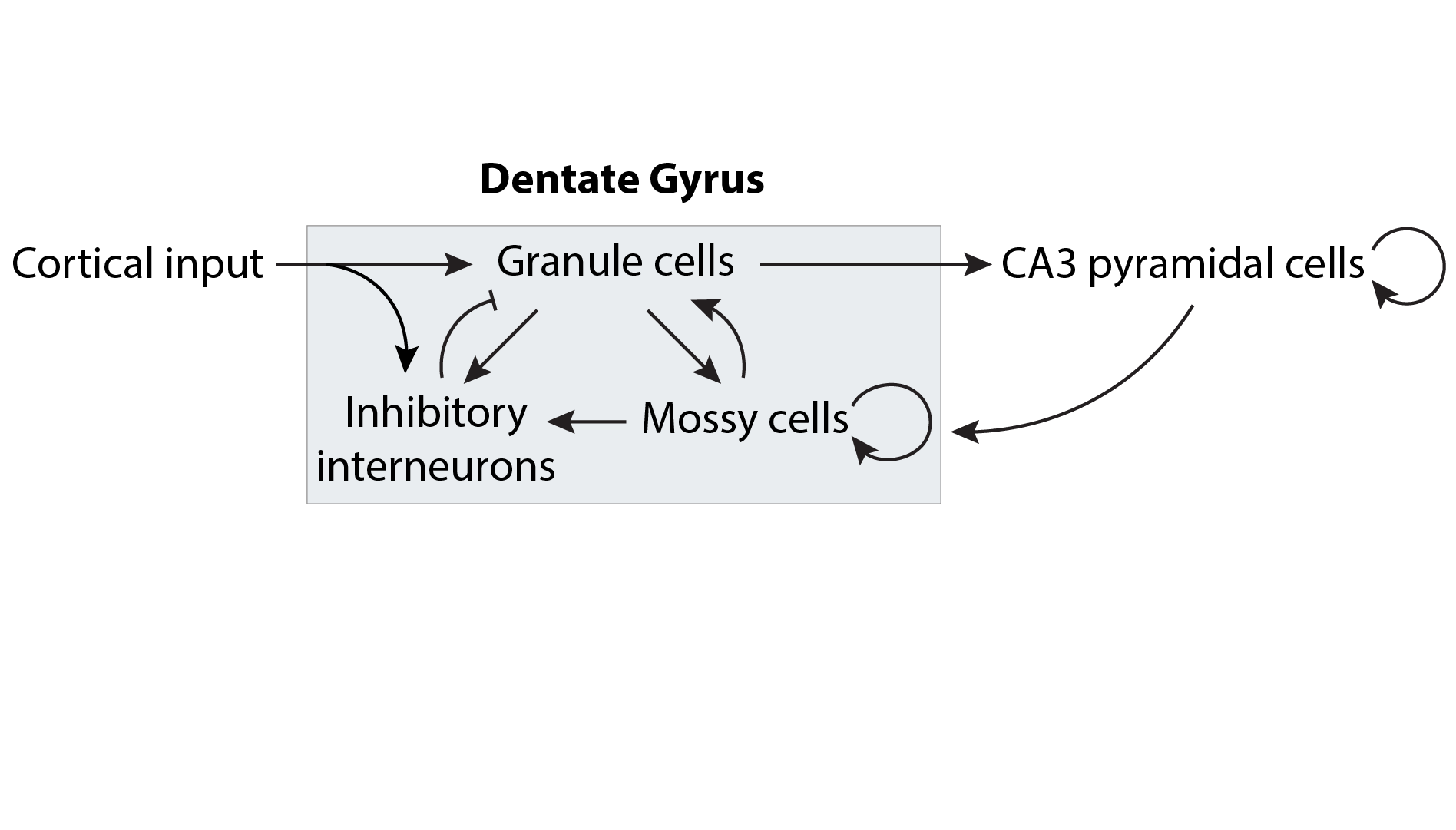
Modelling the dynamics of learning in hippocampal circuits
The hippocampus has a well-characterized anatomical connectivity and encodes many known behavioral features (particularly with regard to spatial memory), but there are still many unknowns regarding how different cell types dynamically interact to coordinate learning in the circuit.
We study this problem by building simplified circuit models of different parts of the hippocampus (e.g. the dentate gyrus) with realistic proportions of different cell types and connectivity motifs, and probing how each network element contributes to the neuronal dynamics and information processing within the circuit.